- QATestLab Blog >
- QA Management >
- Risk Identification Methods In Software Testing
Risk Identification Methods In Software Testing
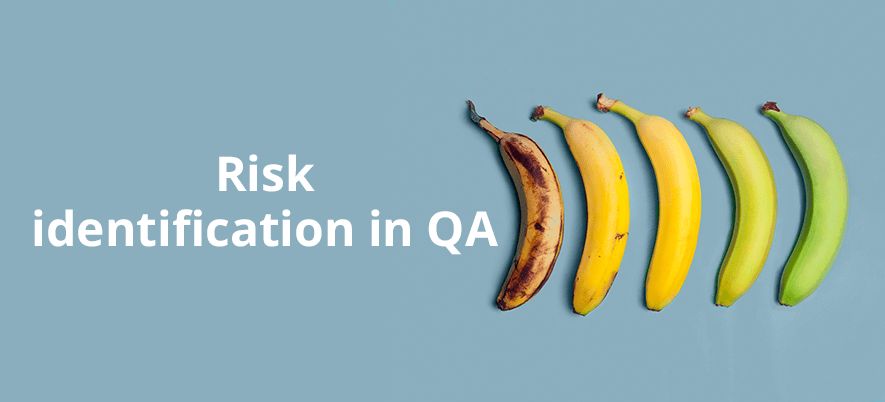
Fault distribution is very uneven for the majority of software, not depending on their size, functionality, implementation language and other features.
Much empirical evidence has accumulated over the years to support the so-called 80:20 principle. It states that 20% of the software elements are answerable for 80% of the troubles.
Such problematic elements may commonly be described by specific estimation properties about their design, size, complexity, change history.
Because of the uneven fault distribution among software elements, there is a huge need for risk identification methods to analyze these estimation data so that inspection, software testing and other quality assurance activities can be concentrated on such potentially high-defect elements.
There are several risk detecting methods:
- tree-based modeling
- traditional statistical analysis methods
- neural networks
- learning algorithms
- pattern matching methods
- principal component and discriminant analysis
These methods can be described by such features as:
- exactness
- presence of tool support
- ease of result interpretation
- simplicity
- stability
- creative info
- early presence
- manual for quality betterment
Correct risk detecting methods may be picked to fit specific application environments with the goal to detect high-risk software elements for focused inspection and software testing.
Learn more from QATestLab
Related Posts:
- How to Identify and Manage Software Testing Risks?
- VivaTech 2025: Wearable AI, Next-Gen Robotics, and Assistive Accessibility Devices
- Inside Shoptalk Europe 2025: Hunting Retail AI InsightsÂ
About Article Author
view more articles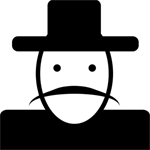
No Comments Yet!
You can be the one to start a conversation.